What is autonomous cars? Autonomous cars are self-driving vehicles that use artificial intelligence (AI) and sensors to navigate and operate without human intervention, using high-resolution cameras and lidars that detect what happens in the car's immediate surroundings. They have the potential to revolutionize transportation by improving safety, efficiency, and accessibility.
Papers and Code
Jan 29, 2025
Abstract:New challenges on transport systems are emerging due to the advances that the current paradigm is experiencing. The breakthrough of the autonomous car brings concerns about ride comfort, while the pollution concerns have arisen in recent years. In the model of automated automobiles, drivers are expected to become passengers, so, they will be more prone to suffer from ride discomfort or motion sickness. Conversely, the eco-driving implications should not be set aside because of the influence of pollution on climate and people's health. For that reason, a joint assessment of the aforementioned points would have a positive impact. Thus, this work presents a self-organised map-based solution to assess ride comfort features of individuals considering their driving style from the viewpoint of eco-driving. For this purpose, a previously acquired dataset from an instrumented car was used to classify drivers regarding the causes of their lack of ride comfort and eco-friendliness. Once drivers are classified regarding their driving style, natural-language-based recommendations are proposed to increase the engagement with the system. Hence, potential improvements of up to the 57.7% for ride comfort evaluation parameters, as well as up to the 47.1% in greenhouse-gasses emissions are expected to be reached.
Via
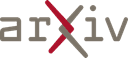
Jan 29, 2025
Abstract:Advanced driving assistance systems (ADAS) are primarily designed to increase driving safety and reduce traffic congestion without paying too much attention to passenger comfort or motion sickness. However, in view of autonomous cars, and taking into account that the lack of comfort and motion sickness increase in passengers, analysis from a comfort perspective is essential in the future car investigation. The aim of this work is to study in detail how passenger's comfort evaluation parameters vary depending on the driving style, car or road. The database used has been developed by compiling the accelerations suffered by passengers when three drivers cruise two different vehicles on different types of routes. In order to evaluate both comfort and motion sickness, first, the numerical values of the main comfort evaluation variables reported in the literature have been analyzed. Moreover, a complementary statistical analysis of probability density and a power spectral analysis are performed. Finally, quantitative results are compared with passenger qualitative feedback. The results show the high dependence of comfort evaluation variables' value with the road type. In addition, it has been demonstrated that the driving style and vehicle dynamics amplify or attenuate those values. Additionally, it has been demonstrated that contributions from longitudinal and lateral accelerations have a much greater effect in the lack of comfort than vertical ones. Finally, based on the concrete results obtained, a new experimental campaign is proposed.
Via
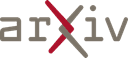
Jan 24, 2025
Abstract:This article proposes an active-learning-based adaptive trajectory tracking control method for autonomous ground vehicles to compensate for modeling errors and unmodeled dynamics. The nominal vehicle model is decoupled into lateral and longitudinal subsystems, which are augmented with online Gaussian Processes (GPs), using measurement data. The estimated mean functions of the GPs are used to construct a feedback compensator, which, together with an LPV state feedback controller designed for the nominal system, gives the adaptive control structure. To assist exploration of the dynamics, the paper proposes a new, dynamic active learning method to collect the most informative samples to accelerate the training process. To analyze the performance of the overall learning tool-chain provided controller, a novel iterative, counterexample-based algorithm is proposed for calculating the induced L2 gain between the reference trajectory and the tracking error. The analysis can be executed for a set of possible realizations of the to-be-controlled system, giving robust performance certificate of the learning method under variation of the vehicle dynamics. The efficiency of the proposed control approach is shown on a high-fidelity physics simulator and in real experiments using a 1/10 scale F1TENTH electric car.
* Submitted to IEEE Transactions on Control Systems Technology
Via
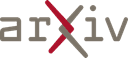
Jan 19, 2025
Abstract:3D car modeling is crucial for applications in autonomous driving systems, virtual and augmented reality, and gaming. However, due to the distinctive properties of cars, such as highly reflective and transparent surface materials, existing methods often struggle to achieve accurate 3D car reconstruction.To address these limitations, we propose Car-GS, a novel approach designed to mitigate the effects of specular highlights and the coupling of RGB and geometry in 3D geometric and shading reconstruction (3DGS). Our method incorporates three key innovations: First, we introduce view-dependent Gaussian primitives to effectively model surface reflections. Second, we identify the limitations of using a shared opacity parameter for both image rendering and geometric attributes when modeling transparent objects. To overcome this, we assign a learnable geometry-specific opacity to each 2D Gaussian primitive, dedicated solely to rendering depth and normals. Third, we observe that reconstruction errors are most prominent when the camera view is nearly orthogonal to glass surfaces. To address this issue, we develop a quality-aware supervision module that adaptively leverages normal priors from a pre-trained large-scale normal model.Experimental results demonstrate that Car-GS achieves precise reconstruction of car surfaces and significantly outperforms prior methods. The project page is available at https://lcc815.github.io/Car-GS.
Via
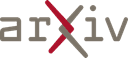
Jan 21, 2025
Abstract:Video encoders optimize compression for human perception by minimizing reconstruction error under bit-rate constraints. In many modern applications such as autonomous driving, an overwhelming majority of videos serve as input for AI systems performing tasks like object recognition or segmentation, rather than being watched by humans. It is therefore useful to optimize the encoder for a downstream task instead of for perceptual image quality. However, a major challenge is how to combine such downstream optimization with existing standard video encoders, which are highly efficient and popular. Here, we address this challenge by controlling the Quantization Parameters (QPs) at the macro-block level to optimize the downstream task. This granular control allows us to prioritize encoding for task-relevant regions within each frame. We formulate this optimization problem as a Reinforcement Learning (RL) task, where the agent learns to balance long-term implications of choosing QPs on both task performance and bit-rate constraints. Notably, our policy does not require the downstream task as an input during inference, making it suitable for streaming applications and edge devices such as vehicles. We demonstrate significant improvements in two tasks, car detection, and ROI (saliency) encoding. Our approach improves task performance for a given bit rate compared to traditional task agnostic encoding methods, paving the way for more efficient task-aware video compression.
Via
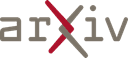
Jan 16, 2025
Abstract:In real-world sequential decision making tasks like autonomous driving, robotics, and healthcare, learning from observed state-action trajectories is critical for tasks like imitation, classification, and clustering. For example, self-driving cars must replicate human driving behaviors, while robots and healthcare systems benefit from modeling decision sequences, whether or not they come from expert data. Existing trajectory encoding methods often focus on specific tasks or rely on reward signals, limiting their ability to generalize across domains and tasks. Inspired by the success of embedding models like CLIP and BERT in static domains, we propose a novel method for embedding state-action trajectories into a latent space that captures the skills and competencies in the dynamic underlying decision-making processes. This method operates without the need for reward labels, enabling better generalization across diverse domains and tasks. Our contributions are threefold: (1) We introduce a trajectory embedding approach that captures multiple abilities from state-action data. (2) The learned embeddings exhibit strong representational power across downstream tasks, including imitation, classification, clustering, and regression. (3) The embeddings demonstrate unique properties, such as controlling agent behaviors in IQ-Learn and an additive structure in the latent space. Experimental results confirm that our method outperforms traditional approaches, offering more flexible and powerful trajectory representations for various applications. Our code is available at https://github.com/Erasmo1015/vte.
* AAMAS 2025
Via
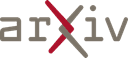
Jan 17, 2025
Abstract:Radar and LiDAR have been widely used in autonomous driving as LiDAR provides rich structure information, and radar demonstrates high robustness under adverse weather. Recent studies highlight the effectiveness of fusing radar and LiDAR point clouds. However, challenges remain due to the modality misalignment and information loss during feature extractions. To address these issues, we propose a 4D radar-LiDAR framework to mutually enhance their representations. Initially, the indicative features from radar are utilized to guide both radar and LiDAR geometric feature learning. Subsequently, to mitigate their sparsity gap, the shape information from LiDAR is used to enrich radar BEV features. Extensive experiments on the View-of-Delft (VoD) dataset demonstrate our approach's superiority over existing methods, achieving the highest mAP of 71.76% across the entire area and 86.36\% within the driving corridor. Especially for cars, we improve the AP by 4.17% and 4.20% due to the strong indicative features and symmetric shapes.
* Accepted by ICASSP 2025
Via
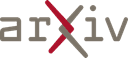
Jan 13, 2025
Abstract:Modeling car-following behavior is essential for traffic simulation, analyzing driving patterns, and understanding complex traffic flows with varying levels of autonomous vehicles. Traditional models like the Safe Distance Model and Intelligent Driver Model (IDM) require precise parameter calibration and often lack generality due to simplified assumptions about driver behavior. While machine learning and deep learning methods capture complex patterns, they require large labeled datasets. Foundation models provide a more efficient alternative. Pre-trained on vast, diverse time series datasets, they can be applied directly to various tasks without the need for extensive re-training. These models generalize well across domains, and with minimal fine-tuning, they can be adapted to specific tasks like car-following behavior prediction. In this paper, we apply Chronos, a state-of-the-art public time series foundation model, to analyze car-following behavior using the Open ACC dataset. Without fine-tuning, Chronos outperforms traditional models like IDM and Exponential smoothing with trend and seasonality (ETS), and achieves similar results to deep learning models such as DeepAR and TFT, with an RMSE of 0.60. After fine-tuning, Chronos reduces the error to an RMSE of 0.53, representing a 33.75% improvement over IDM and a 12-37% reduction compared to machine learning models like ETS and deep learning models including DeepAR, WaveNet, and TFT. This demonstrates the potential of foundation models to significantly advance transportation research, offering a scalable, adaptable, and highly accurate approach to predicting and simulating car-following behaviors.
Via
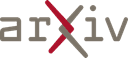
Jan 06, 2025
Abstract:The Lane Keeping Assist (LKA) system has become a standard feature in recent car models. While marketed as providing auto-steering capabilities, the system's operational characteristics and safety performance remain underexplored, primarily due to a lack of real-world testing and comprehensive data. To fill this gap, we extensively tested mainstream LKA systems from leading U.S. automakers in Tampa, Florida. Using an innovative method, we collected a comprehensive dataset that includes full Controller Area Network (CAN) messages with LKA attributes, as well as video, perception, and lateral trajectory data from a high-quality front-facing camera equipped with advanced vision detection and trajectory planning algorithms. Our tests spanned diverse, challenging conditions, including complex road geometry, adverse weather, degraded lane markings, and their combinations. A vision language model (VLM) further annotated the videos to capture weather, lighting, and traffic features. Based on this dataset, we present an empirical overview of LKA's operational features and safety performance. Key findings indicate: (i) LKA is vulnerable to faint markings and low pavement contrast; (ii) it struggles in lane transitions (merges, diverges, intersections), often causing unintended departures or disengagements; (iii) steering torque limitations lead to frequent deviations on sharp turns, posing safety risks; and (iv) LKA systems consistently maintain rigid lane-centering, lacking adaptability on tight curves or near large vehicles such as trucks. We conclude by demonstrating how this dataset can guide both infrastructure planning and self-driving technology. In view of LKA's limitations, we recommend improvements in road geometry and pavement maintenance. Additionally, we illustrate how the dataset supports the development of human-like LKA systems via VLM fine-tuning and Chain of Thought reasoning.
Via
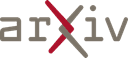
Dec 23, 2024
Abstract:Validating the behavior of autonomous Cyber-Physical Systems (CPS) and Artificial Intelligence (AI) agents, which rely on automated controllers, is an objective of great importance. In recent years, Neural-Network (NN) controllers have been demonstrating great promise. Unfortunately, such learned controllers are often not certified and can cause the system to suffer from unpredictable or unsafe behavior. To mitigate this issue, a great effort has been dedicated to automated verification of systems. Specifically, works in the category of ``black-box testing'' rely on repeated system simulations to find a falsifying counterexample of a system run that violates a specification. As running high-fidelity simulations is computationally demanding, the goal of falsification approaches is to minimize the simulation effort (NN inference queries) needed to return a falsifying example. This often proves to be a great challenge, especially when the tested controller is well-trained. This work contributes a novel falsification approach for autonomous systems under formal specification operating in uncertain environments. We are especially interested in CPS operating in rich, semantically-defined, open environments, which yield high-dimensional, simulation-dependent sensor observations. Our approach introduces a novel reformulation of the falsification problem as the problem of planning a trajectory for a ``meta-system,'' which wraps and encapsulates the examined system; we call this approach: meta-planning. This formulation can be solved with standard sampling-based motion-planning techniques (like RRT) and can gradually integrate domain knowledge to improve the search. We support the suggested approach with an experimental study on falsification of an obstacle-avoiding autonomous car with a NN controller, where meta-planning demonstrates superior performance over alternative approaches.
Via
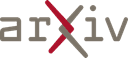